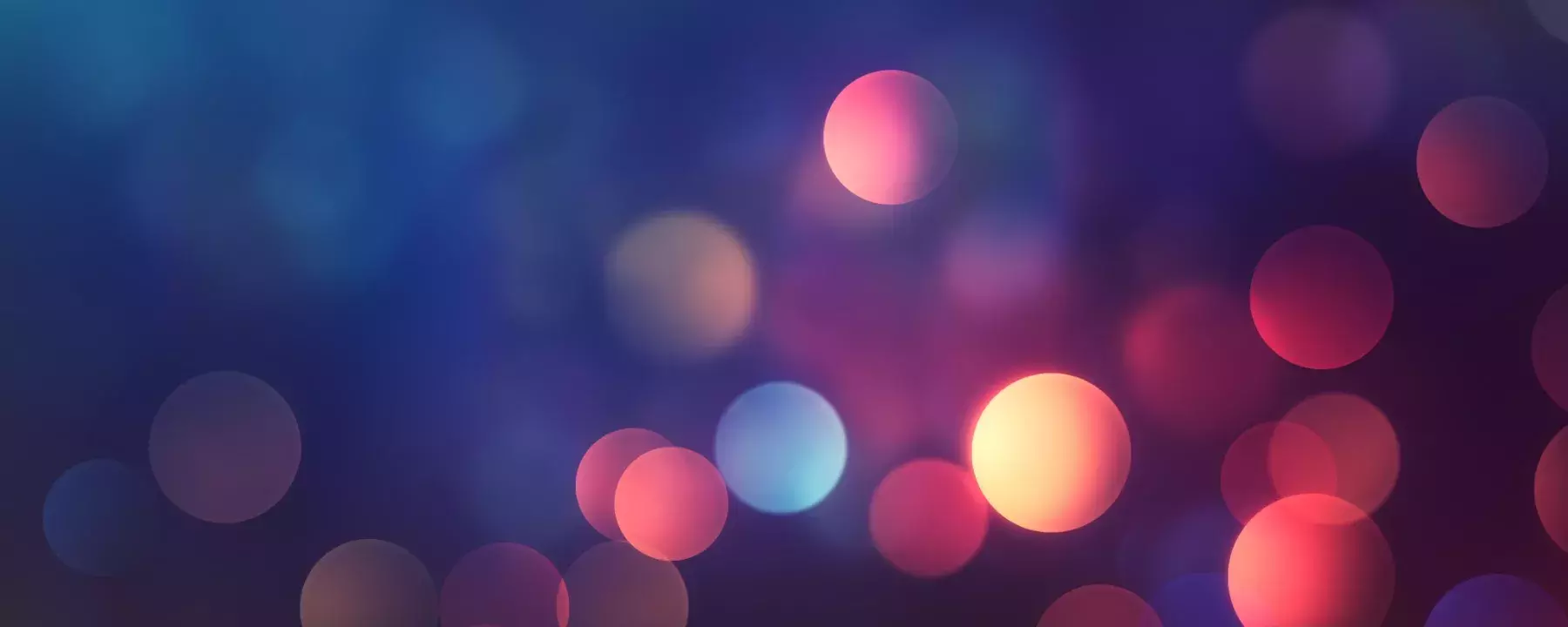
Resources and insights
- Home
- Our thinking
Showing 487 search results
Spotlight: customer experience during the cost of living crisis
When reality bites, we find ways to bite back
We recently held another stimulating discussion in our series of events tracking the cost-of-living crisis, its effects and what actions businesses are taking. Read the article to find out what these are, and learn about current trends.
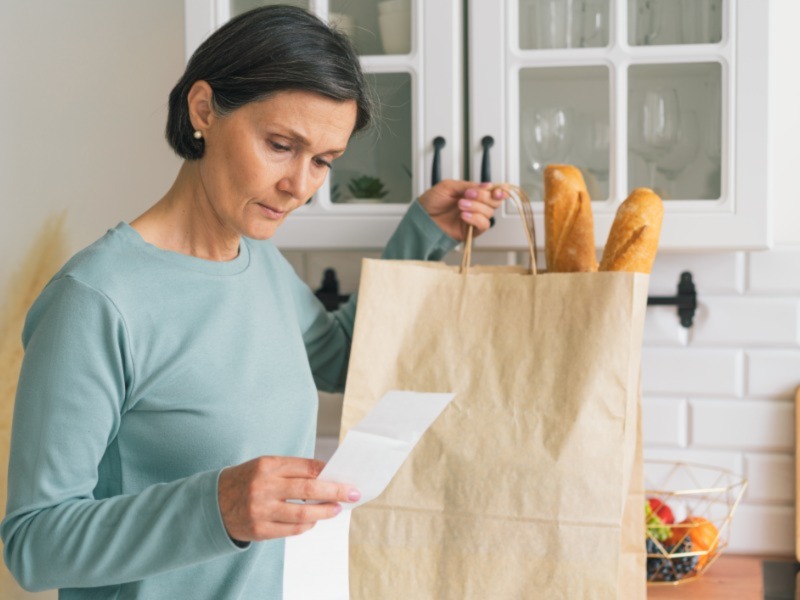